Relative risk: Difference between revisions
John Quiggin (talk | contribs) Clarified on logistic regression |
m fix spacing around math (via WP:JWB) |
||
(295 intermediate revisions by more than 100 users not shown) | |||
Line 1: | Line 1: | ||
{{short description|Measure of association used in epidemiology}} |
|||
In [[statistics]] and mathematical [[epidemiology]], '''relative risk (RR)''' is the risk of an event (or of developing a disease) relative to exposure. Relative risk is a [[ratio]] of the [[probability]] of the event occurring in the exposed group versus the control (non-exposed) group. |
|||
[[File:Illustration of risk reduction.svg|alt=Illustration of two groups: one exposed to treatment, and one unexposed. Exposed group has smaller risk of adverse outcome, with RR = 4/8 = 0.5.|thumb|The group exposed to treatment (left) has half the risk (RR = 4/8 = 0.5) of an adverse outcome (black) compared to the unexposed group (right).]] |
|||
The '''relative risk (RR)''' or '''risk ratio''' is the ratio of the [[probability]] of an outcome in an exposed group to the probability of an outcome in an unexposed group. Together with [[risk difference]] and [[odds ratio]], relative risk measures the association between the exposure and the outcome.<ref name="pmid14695382">{{cite journal | vauthors = Sistrom CL, Garvan CW | title = Proportions, odds, and risk | journal = Radiology | volume = 230 | issue = 1 | pages = 12–9 | date = January 2004 | pmid = 14695382 | doi = 10.1148/radiol.2301031028 }}</ref> |
|||
==Statistical use and meaning== |
|||
:<math>RR= \frac {p_\mathrm{exposed}}{p_\mathrm{control}} </math> |
|||
Relative risk is used in the statistical analysis of the data of [[Ecological study|ecological]], [[Cohort study|cohort]], medical and intervention studies, to estimate the strength of the association between exposures (treatments or risk factors) and outcomes.<ref name=":2">{{Cite book|last=Carneiro, Ilona.|url=https://www.worldcat.org/oclc/773348873|title=Introduction to epidemiology|date=2011|publisher=Open University Press|others=Howard, Natasha.|isbn=978-0-335-24462-1|edition=2nd|location=Maidenhead, Berkshire|pages=27|oclc=773348873}}</ref> Mathematically, it is the incidence rate of the outcome in the exposed group, <math>I_e</math>, divided by the rate of the unexposed group, <math>I_u</math>.<ref>{{Cite book|last=Bruce, Nigel, 1955-|url=https://www.worldcat.org/oclc/992438133|title=Quantitative methods for health research : a practical interactive guide to epidemiology and statistics|others=Pope, Daniel, 1969-, Stanistreet, Debbi, 1963-|date=29 November 2017|isbn=978-1-118-66526-8|edition=Second|location=Hoboken, NJ|pages=199|oclc=992438133}}</ref> As such, it is used to compare the risk of an adverse outcome when receiving a medical treatment versus no treatment (or placebo), or for environmental risk factors. For example, in a study examining the effect of the drug apixaban on the occurrence of thromboembolism, 8.8% of placebo-treated patients experienced the disease, but only 1.7% of patients treated with the drug did, so the relative risk is .19 (1.7/8.8): patients receiving apixaban had 19% the disease risk of patients receiving the placebo.<ref>{{Cite book|last=Motulsky, Harvey|url=https://www.worldcat.org/oclc/1006531983|title=Intuitive biostatistics : a nonmathematical guide to statistical thinking|year=2018|isbn=978-0-19-064356-0|edition=Fourth|location=New York|pages=266|oclc=1006531983}}</ref> In this case, apixaban is a [[protective factor]] rather than a [[risk factor]], because it reduces the risk of disease. |
|||
Assuming the causal effect between the exposure and the outcome, values of relative risk can be interpreted as follows:<ref name=":2" /> |
|||
For example, if the [[probability]] of developing lung cancer among smokers was 20% and among non-smokers 10%, then the relative risk of cancer associated with smoking would be 2. Smokers would be twice as likely as non-smokers to develop lung cancer. |
|||
* RR = 1 means that exposure does not affect the outcome |
|||
* RR < 1 means that the risk of the outcome is decreased by the exposure, which is a "[[protective factor]]" |
|||
* RR > 1 means that the risk of the outcome is increased by the exposure, which is a "[[risk factor]]" |
|||
As always, correlation does not mean causation; the causation could be reversed, or they could both be caused by a common [[Confounding|confounding variable]]. The relative risk of having cancer when in the hospital versus at home, for example, would be greater than 1, but that is because having cancer causes people to go to the hospital. |
|||
== Statistical use and meaning == |
|||
== Usage in reporting == |
|||
Relative risk is used frequently in the statistical analysis of binary outcomes where the outcome of interest has relatively low probability. It is thus often suited to [[clinical trial]] data, where it is used to compare the risk of developing a disease, in people receiving a new medical treatment versus people receiving an established (standard of care) treatment or a [[placebo]]. It is particularly attractive because it can be calculated by hand in the simple case, but is also susceptible to [[regression analysis|regression modelling]], typically in a [[Poisson regression]] framework. |
|||
Relative risk is commonly used to present the results of randomized controlled trials.<ref>{{cite journal | vauthors = Nakayama T, Zaman MM, Tanaka H | title = Reporting of attributable and relative risks, 1966-97 | journal = Lancet | volume = 351 | issue = 9110 | pages = 1179 | date = April 1998 | pmid = 9643696 | doi = 10.1016/s0140-6736(05)79123-6 | s2cid = 28195147 }}</ref> This can be problematic if the relative risk is presented without the absolute measures, such as [[absolute risk]], or risk difference.<ref>{{cite journal | vauthors = Noordzij M, van Diepen M, Caskey FC, Jager KJ | title = Relative risk versus absolute risk: one cannot be interpreted without the other | journal = Nephrology, Dialysis, Transplantation | volume = 32 | issue = suppl_2 | pages = ii13–ii18 | date = April 2017 | pmid = 28339913 | doi = 10.1093/ndt/gfw465 | doi-access = free }}</ref> In cases where the base rate of the outcome is low, large or small values of relative risk may not translate to significant effects, and the importance of the effects to the public health can be overestimated. Equivalently, in cases where the base rate of the outcome is high, values of the relative risk close to 1 may still result in a significant effect, and their effects can be underestimated. Thus, presentation of both absolute and relative measures is recommended.<ref>{{cite journal | vauthors = Moher D, Hopewell S, Schulz KF, Montori V, Gøtzsche PC, Devereaux PJ, Elbourne D, Egger M, Altman DG | title = CONSORT 2010 explanation and elaboration: updated guidelines for reporting parallel group randomised trials | journal = BMJ | volume = 340 | pages = c869 | date = March 2010 | pmid = 20332511 | pmc = 2844943 | doi = 10.1136/bmj.c869 }}</ref> |
|||
== Inference == |
|||
*A relative risk of 1 means there is no difference in risk between the two groups. |
|||
Relative risk can be estimated from a 2×2 [[contingency table]]: |
|||
*A RR of < 1 means the event is less likely to occur in the experimental group than in the control group. |
|||
{| class="wikitable" |
|||
*A RR of > 1 means the event is more likely to occur in the experimental group than in the control group. |
|||
! rowspan="2" | |
|||
! colspan="2" |Group |
|||
|- |
|||
! Intervention (I) |
|||
![[Scientific control|Control]] (C) |
|||
|- |
|||
| Events (E) |
|||
| IE |
|||
| CE |
|||
|- |
|||
| Non-events (N) |
|||
| IN |
|||
| CN |
|||
|} |
|||
The point estimate of the relative risk is |
|||
The log of relative risk is usually taken to have a sampling distribution that is approximately normal. This permits the construction of a [[confidence interval]] which is symmetric around <math>\log(RR)</math>, i.e. |
|||
:<math> |
:<math>RR = \frac{IE/(IE + IN)}{CE/(CE + CN)} = \frac{IE(CE + CN)}{CE(IE + IN)}.</math> |
||
The sampling distribution of the <math>\log(RR)</math> is closer to normal than the distribution of RR,<ref>{{cite web|url=https://www.stata.com/support/faqs/stat/2deltameth.html|title=Standard errors, confidence intervals, and significance tests|work=StataCorp LLC}}</ref> with standard error |
|||
where <math>z_\alpha</math> is the z-score for the chosen level of [[statistical significance|significance]]. The antilog can be taken of the two bounds of the log-CI, giving the high and low bounds for an asymmetric confidence interval around the relative risk. |
|||
:<math>SE(\log(RR)) = \sqrt{\frac{IN}{IE(IE + IN)} + \frac{CN}{CE(CE + CN)}}.</math> |
|||
=== Association with odds ratio === |
|||
Relative risk is different from the [[odds ratio]], although it asymptotically approaches it for small probabilities. In fact, the odds ratio has much wider use in statistics, since [[logistic regression]], often associated with [[clinical trial]]s, works with the log of the odds ratio, not relative risk. Because the log of the odds ratio is estimated as a linear function of the explanatory vaiables, the estimated odds ratio for 70-year-olds and 60-year-olds associated with type of treatment would be the same in a logistic regression models where the outcome is associated with drug and age, although the relative risk might be significantly different. In cases like this, statistical models of the odds ratio often reflect the underlying mechanisms more effectively. |
|||
The <math>1 - \alpha</math> confidence interval for the <math>\log(RR)</math> is then |
|||
Since relative risk is a more intuitive measure of effectiveness, the distinction is important especially in cases of medium to high probabilities. If action A carries a risk of 99.9% and action B a risk of 99.0% then the relative risk is just over 1, while the odds associated with action A are almost 10 times higher than the odds with B. |
|||
:<math>CI_{1 - \alpha}(\log(RR)) = \log(RR)\pm SE(\log(RR))\times z_\alpha,</math> |
|||
In medical research, the [[odds ratio]] is favoured for [[case-control study|case-control studies]] and [[retrospective study|retrospective studies]]. Relative risk is used in [[randomized controlled trial]]s and [[cohort study|cohort studies]].<ref>Medical University of South Carolina. [http://www.musc.edu/dc/icrebm/oddsratio.html Odds ratio versus relative risk]. Accessed on: [[September 8]], [[2005]].</ref> |
|||
where <math>z_\alpha</math> is the [[standard score]] for the chosen level of [[statistical significance|significance]].<ref name=":1">{{Cite book|title=Epidemiology : beyond the basics|last1=Szklo|first1=Moyses|last2=Nieto|first2=F. Javier|publisher=Jones & Bartlett Learning|year=2019|isbn=9781284116595|edition=4th.|location=Burlington, Massachusetts|pages=488|oclc=1019839414}}</ref><ref>{{Cite journal|last1=Katz|first1=D.|last2=Baptista|first2=J.|last3=Azen|first3=S. P.|last4=Pike|first4=M. C.|date=1978|title=Obtaining Confidence Intervals for the relative risk in Cohort Studies|journal=Biometrics|volume=34|issue=3|pages=469–474|doi=10.2307/2530610|jstor=2530610}}</ref> To find the confidence interval around the RR itself, the two bounds of the above confidence interval can be [[Exponentiation|exponentiated]].<ref name=":1" /> |
|||
In statistical modelling, approaches like [[poisson regression]] (for counts of events per unit exposure) have relative risk interpretations: the estimated effect of an explanatory variable is multiplicative on the rate, and thus leads to a risk ratio or relative risk. [[Logistic regression]] (for binary outcomes, or counts of successes out of a number of trials) must be interpreted in odds-ratio terms: the effect of an explanatory variable is multiplicative on the odds and thus leads to an odds ratio. |
|||
In regression models, the exposure is typically included as an [[dummy variable (statistics)|indicator variable]] along with other factors that may affect risk. The relative risk is usually reported as calculated for the [[mean]] of the sample values of the explanatory variables.{{cn|date=May 2023}} |
|||
==Size of relative risk and relevance== |
|||
In the hypothesis testing framework, the null hypothesis is that RR=1 (the putative risk factor has no effect). The null hypothesis can be rejected in favor of the alternative hypothesis of that the factor in question does affect risk if the confidence interval for RR excludes 1. |
|||
== Comparison to the odds ratio == |
|||
Critics of the standard approach, notably including [[John Brignell]] and [[Steven Milloy]], believe published studies suffer from unduly high [[type I error]] rates, and have argued for an additional requirement that the point estimate of RR should exceed 2 [http://www.numberwatch.co.uk/RR.htm] [http://www.numberwatch.co.uk/2005%20November.htm#RR] [http://www.junkscience.com/news/sws/sws-chapter2.html] (or, if risks are reduced, be below 0.5) and have cited a variety of statements by statisticians and others supporting this view. The issue has arisen particularly in relation to debates about the effects of [[passive smoking]], where the effect size appears to be small (relative to smoking), and exposure levels are difficult to quantify in the affected population. |
|||
[[File:Risk Ratio vs Odds Ratio.svg|thumb|Risk ratio vs odds ratio]] |
|||
The relative risk is different from the [[odds ratio]], although the odds ratio asymptotically approaches the relative risk for small probabilities of outcomes. If IE is substantially smaller than ''IN'', then IE/(IE + IN) <math>\scriptstyle\approx</math> IE/IN. Similarly, if CE is much smaller than CN, then CE/(CN + CE) <math>\scriptstyle\approx</math> CE/CN. Thus, under [[the rare disease assumption]] |
|||
:<math> RR = \frac{IE(CE + CN)}{CE(IE + IN)} \approx \frac{IE \cdot CN}{IN \cdot CE} = OR.</math> |
|||
In support of this claim, it may be observed that, if the base level of risk is low, a small proportionate increase in risk may be of little practical signifance. (In the case of lung cancer, however, the base risk is substantial). |
|||
In practice the [[odds ratio]] is commonly used for [[case-control study|case-control studies]], as the relative risk cannot be estimated.<ref name="pmid14695382" /> |
|||
In addition, if estimates are biased by the exclusion of relevant factors, the likelihood of a spurious finding of significance is greater if the estimated RR is close to 1. In his paper "Why Most Published Research Findings Are False" [http://medicine.plosjournals.org/perlserv/?request=get-document&doi=10.1371%2Fjournal.pmed.0020124], John Ioannidis writes "The smaller the effect sizes in a scientific field, the less likely the research findings are to be true. [...] research findings are more likely true in scientific fields with [...] relative risks 3–20 [...], than in scientific fields where postulated effects are small [...] (relative risks 1.1–1.5)." "if the majority of true genetic or nutritional determinants of complex diseases confer relative risks less than 1.05, genetic or nutritional epidemiology would be largely utopian endeavors." |
|||
In fact, the odds ratio has much more common use in statistics, since [[logistic regression]], often associated with [[clinical trial]]s, works with the log of the odds ratio, not relative risk. Because the (natural log of the) odds of a record is estimated as a linear function of the explanatory variables, the estimated odds ratio for 70-year-olds and 60-year-olds associated with the type of treatment would be the same in logistic regression models where the outcome is associated with drug and age, although the relative risk might be significantly different.{{cn|date=August 2023}} |
|||
However, a blanket requirement that RR>2, taking no account of base rates or sample size, is a fairly crude solution to the problem, and one that appears unduly favorable to opponents of regulation. For this reason, most statisticians continue to use the standard hypothesis testing framework, though with more caution than would be indicated by a standard textbook account. |
|||
Since relative risk is a more intuitive measure of effectiveness, the distinction is important especially in cases of medium to high probabilities. If action A carries a risk of 99.9% and action B a risk of 99.0% then the relative risk is just over 1, while the odds associated with action A are more than 10 times higher than the odds with B.{{cn|date=August 2023}} |
|||
===Statistical significance (confidence) and relative risk=== |
|||
Whether a given relative risk can be considered [[statistical significance|statistically significant]] is dependent on the relative difference between the conditions compared, the amount of measurement and the noise associated with the measurement (of the events considered). In other words, the confidence one has, in a given relative risk being non-random (i.e. it is not a consequence of [[chance]]), depends on the [[signal-to-noise ratio]] and the sample size. |
|||
In statistical modelling, approaches like [[Poisson regression]] (for counts of events per unit exposure) have relative risk interpretations: the estimated effect of an explanatory variable is multiplicative on the rate and thus leads to a relative risk. [[Logistic regression]] (for binary outcomes, or counts of successes out of a number of trials) must be interpreted in odds-ratio terms: the effect of an explanatory variable is multiplicative on the odds and thus leads to an odds ratio.{{cn|date=August 2023}} |
|||
Expressed mathematically, the confidence that a result is not by random chance is given by the following formula by Sackett<ref>Sackett DL. Why randomized controlled trials fail but needn't: 2. Failure to employ physiological statistics, or the only formula a clinician-trialist is ever likely to need (or understand!). CMAJ. 2001 Oct 30;165(9):1226-37. PMID 11706914. [http://www.cmaj.ca/cgi/content/full/165/9/1226 Free Full Text].</ref>: |
|||
== Bayesian interpretation == |
|||
<math>confidence = \frac{signal}{noise} \times \sqrt{samplesize}</math> |
|||
For clarity, the above forumla is presented in tabular form below. |
|||
'''Dependence of confidence with noise, signal and sample size (tabular form)''' |
|||
{| border="1" cellpadding="2" |
|||
!Parameter |
|||
!Parameter increases |
|||
!Parameter decreases |
|||
|- |
|||
|Noise |
|||
|Confidence decreases |
|||
|Confidence increases |
|||
|- |
|||
|Signal |
|||
|Confidence increases |
|||
|Confidence decreases |
|||
|- |
|||
|Sample size |
|||
|Confidence increases |
|||
|Confidence decreases |
|||
|} |
|||
We could assume a disease noted by <math>D</math>, and no disease noted by <math>\neg D</math>, exposure noted by <math>E</math>, and no exposure noted by <math>\neg E</math>. The relative risk can be written as |
|||
:<math>RR = \frac {P(D\mid E)}{P(D\mid \neg E)} = \frac {P(E\mid D)/P(\neg E\mid D)}{P(E)/P(\neg E)}. </math> |
|||
In words, the dependence of confidence is high if the noise is low and/or the sample size is large and/or the effect size (signal) is large. The confidence of a relative risk value (and its associated confidence interval) is ''not'' dependent on effect size alone. If the sample size is large and the noise is low a small effect size can be measured with great confidence. Whether a small effect size is considered important is dependent on the context of the events compared. |
|||
This way the relative risk can be interpreted in Bayesian terms as the posterior ratio of the exposure (i.e. after seeing the disease) normalized by the prior ratio of exposure.<ref>{{cite book|title=Statistical Methods in Medical Research |vauthors=[[Peter Armitage (statistician)|Armitage P]], Berry G, Matthews JN|journal=[[Proceedings of the Royal Society of Medicine]] |editor1-first=P|editor1-last=Armitage|editor2-first=G|editor2-last=Berry|editor3-first=J.N.S|editor3-last=Matthews|date=2002|volume=64|issue=11|page=1168|publisher=Blackwell Science Ltd|isbn=978-0-470-77366-6|edition=Fourth|doi=10.1002/9780470773666|pmc=1812060}}</ref> If the posterior ratio of exposure is similar to that of the prior, the effect is approximately 1, indicating no association with the disease, since it didn't change beliefs of the exposure. If on the other hand, the posterior ratio of exposure is smaller or higher than that of the prior ratio, then the disease has changed the view of the exposure danger, and the magnitude of this change is the relative risk. |
|||
In medicine, small effect sizes (reflected by small relative risk values) are usually considered clinically relevant (if there is great confidence in them) and are frequently used to guide treatment decisions. A relative risk of 1.10 may seem very small, but over a large number of patients will make a noticeable difference. Whether a given treatment is considered a worthy endeavour is dependent on the risks, benefits and costs. |
|||
== |
==Numerical example== |
||
{{RCT risk reduction example}} |
|||
*[[Confidence interval]] |
|||
*[[Odds ratio]] |
|||
*[[Hazard ratio]] |
|||
*[[Number needed to treat]] (NNT) |
|||
*[[Number needed to harm]] (NNH) |
|||
== |
== See also == |
||
{{commons category|Statistics for relative risk}} |
|||
<references/> |
|||
* [[Absolute risk]] |
|||
* [[Relative risk reduction]] |
|||
* [[Base rate fallacy]] |
|||
* [[Cochran–Mantel–Haenszel statistics]] for aggregation of risk ratios across several strata |
|||
* [[Population impact measure]] |
|||
* [[OpenEpi]] |
|||
* [[Rate ratio]] |
|||
== |
== References == |
||
{{reflist}} |
|||
*[http://www.mindspring.com/~hlthdata/ex-rr1.html Relative risk] |
|||
*[http://www.cebm.utoronto.ca/glossary/ EBM glossary] |
|||
== External links == |
|||
* [http://www.medcalc.org/calc/relative_risk.php Relative risk online calculator] |
|||
{{Medical research studies}} |
|||
[[Category: Epidemiology]] |
|||
{{Public health}} |
|||
[[Category: Statistics]] |
|||
{{DEFAULTSORT:Relative Risk}} |
|||
{{statistics-stub}} |
|||
[[Category:Epidemiology]] |
|||
[[Category:Biostatistics]] |
|||
[[Category:Medical statistics]] |
|||
[[Category:Statistical ratios]] |
Latest revision as of 12:47, 4 October 2024
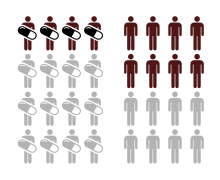
The relative risk (RR) or risk ratio is the ratio of the probability of an outcome in an exposed group to the probability of an outcome in an unexposed group. Together with risk difference and odds ratio, relative risk measures the association between the exposure and the outcome.[1]
Statistical use and meaning
[edit]Relative risk is used in the statistical analysis of the data of ecological, cohort, medical and intervention studies, to estimate the strength of the association between exposures (treatments or risk factors) and outcomes.[2] Mathematically, it is the incidence rate of the outcome in the exposed group, , divided by the rate of the unexposed group, .[3] As such, it is used to compare the risk of an adverse outcome when receiving a medical treatment versus no treatment (or placebo), or for environmental risk factors. For example, in a study examining the effect of the drug apixaban on the occurrence of thromboembolism, 8.8% of placebo-treated patients experienced the disease, but only 1.7% of patients treated with the drug did, so the relative risk is .19 (1.7/8.8): patients receiving apixaban had 19% the disease risk of patients receiving the placebo.[4] In this case, apixaban is a protective factor rather than a risk factor, because it reduces the risk of disease.
Assuming the causal effect between the exposure and the outcome, values of relative risk can be interpreted as follows:[2]
- RR = 1 means that exposure does not affect the outcome
- RR < 1 means that the risk of the outcome is decreased by the exposure, which is a "protective factor"
- RR > 1 means that the risk of the outcome is increased by the exposure, which is a "risk factor"
As always, correlation does not mean causation; the causation could be reversed, or they could both be caused by a common confounding variable. The relative risk of having cancer when in the hospital versus at home, for example, would be greater than 1, but that is because having cancer causes people to go to the hospital.
Usage in reporting
[edit]Relative risk is commonly used to present the results of randomized controlled trials.[5] This can be problematic if the relative risk is presented without the absolute measures, such as absolute risk, or risk difference.[6] In cases where the base rate of the outcome is low, large or small values of relative risk may not translate to significant effects, and the importance of the effects to the public health can be overestimated. Equivalently, in cases where the base rate of the outcome is high, values of the relative risk close to 1 may still result in a significant effect, and their effects can be underestimated. Thus, presentation of both absolute and relative measures is recommended.[7]
Inference
[edit]Relative risk can be estimated from a 2×2 contingency table:
Group | ||
---|---|---|
Intervention (I) | Control (C) | |
Events (E) | IE | CE |
Non-events (N) | IN | CN |
The point estimate of the relative risk is
The sampling distribution of the is closer to normal than the distribution of RR,[8] with standard error
The confidence interval for the is then
where is the standard score for the chosen level of significance.[9][10] To find the confidence interval around the RR itself, the two bounds of the above confidence interval can be exponentiated.[9]
In regression models, the exposure is typically included as an indicator variable along with other factors that may affect risk. The relative risk is usually reported as calculated for the mean of the sample values of the explanatory variables.[citation needed]
Comparison to the odds ratio
[edit]
The relative risk is different from the odds ratio, although the odds ratio asymptotically approaches the relative risk for small probabilities of outcomes. If IE is substantially smaller than IN, then IE/(IE + IN) IE/IN. Similarly, if CE is much smaller than CN, then CE/(CN + CE) CE/CN. Thus, under the rare disease assumption
In practice the odds ratio is commonly used for case-control studies, as the relative risk cannot be estimated.[1]
In fact, the odds ratio has much more common use in statistics, since logistic regression, often associated with clinical trials, works with the log of the odds ratio, not relative risk. Because the (natural log of the) odds of a record is estimated as a linear function of the explanatory variables, the estimated odds ratio for 70-year-olds and 60-year-olds associated with the type of treatment would be the same in logistic regression models where the outcome is associated with drug and age, although the relative risk might be significantly different.[citation needed]
Since relative risk is a more intuitive measure of effectiveness, the distinction is important especially in cases of medium to high probabilities. If action A carries a risk of 99.9% and action B a risk of 99.0% then the relative risk is just over 1, while the odds associated with action A are more than 10 times higher than the odds with B.[citation needed]
In statistical modelling, approaches like Poisson regression (for counts of events per unit exposure) have relative risk interpretations: the estimated effect of an explanatory variable is multiplicative on the rate and thus leads to a relative risk. Logistic regression (for binary outcomes, or counts of successes out of a number of trials) must be interpreted in odds-ratio terms: the effect of an explanatory variable is multiplicative on the odds and thus leads to an odds ratio.[citation needed]
Bayesian interpretation
[edit]We could assume a disease noted by , and no disease noted by , exposure noted by , and no exposure noted by . The relative risk can be written as
This way the relative risk can be interpreted in Bayesian terms as the posterior ratio of the exposure (i.e. after seeing the disease) normalized by the prior ratio of exposure.[11] If the posterior ratio of exposure is similar to that of the prior, the effect is approximately 1, indicating no association with the disease, since it didn't change beliefs of the exposure. If on the other hand, the posterior ratio of exposure is smaller or higher than that of the prior ratio, then the disease has changed the view of the exposure danger, and the magnitude of this change is the relative risk.
Numerical example
[edit]Quantity | Experimental group (E) | Control group (C) | Total |
---|---|---|---|
Events (E) | EE = 15 | CE = 100 | 115 |
Non-events (N) | EN = 135 | CN = 150 | 285 |
Total subjects (S) | ES = EE + EN = 150 | CS = CE + CN = 250 | 400 |
Event rate (ER) | EER = EE / ES = 0.1, or 10% | CER = CE / CS = 0.4, or 40% | — |
Variable | Abbr. | Formula | Value |
---|---|---|---|
Absolute risk reduction | ARR | CER − EER | 0.3, or 30% |
Number needed to treat | NNT | 1 / (CER − EER) | 3.33 |
Relative risk (risk ratio) | RR | EER / CER | 0.25 |
Relative risk reduction | RRR | (CER − EER) / CER, or 1 − RR | 0.75, or 75% |
Preventable fraction among the unexposed | PFu | (CER − EER) / CER | 0.75 |
Odds ratio | OR | (EE / EN) / (CE / CN) | 0.167 |
See also
[edit]
- Absolute risk
- Relative risk reduction
- Base rate fallacy
- Cochran–Mantel–Haenszel statistics for aggregation of risk ratios across several strata
- Population impact measure
- OpenEpi
- Rate ratio
References
[edit]- ^ a b Sistrom CL, Garvan CW (January 2004). "Proportions, odds, and risk". Radiology. 230 (1): 12–9. doi:10.1148/radiol.2301031028. PMID 14695382.
- ^ a b Carneiro, Ilona. (2011). Introduction to epidemiology. Howard, Natasha. (2nd ed.). Maidenhead, Berkshire: Open University Press. p. 27. ISBN 978-0-335-24462-1. OCLC 773348873.
- ^ Bruce, Nigel, 1955- (29 November 2017). Quantitative methods for health research : a practical interactive guide to epidemiology and statistics. Pope, Daniel, 1969-, Stanistreet, Debbi, 1963- (Second ed.). Hoboken, NJ. p. 199. ISBN 978-1-118-66526-8. OCLC 992438133.
{{cite book}}
: CS1 maint: location missing publisher (link) CS1 maint: multiple names: authors list (link) CS1 maint: numeric names: authors list (link) - ^ Motulsky, Harvey (2018). Intuitive biostatistics : a nonmathematical guide to statistical thinking (Fourth ed.). New York. p. 266. ISBN 978-0-19-064356-0. OCLC 1006531983.
{{cite book}}
: CS1 maint: location missing publisher (link) - ^ Nakayama T, Zaman MM, Tanaka H (April 1998). "Reporting of attributable and relative risks, 1966-97". Lancet. 351 (9110): 1179. doi:10.1016/s0140-6736(05)79123-6. PMID 9643696. S2CID 28195147.
- ^ Noordzij M, van Diepen M, Caskey FC, Jager KJ (April 2017). "Relative risk versus absolute risk: one cannot be interpreted without the other". Nephrology, Dialysis, Transplantation. 32 (suppl_2): ii13–ii18. doi:10.1093/ndt/gfw465. PMID 28339913.
- ^ Moher D, Hopewell S, Schulz KF, Montori V, Gøtzsche PC, Devereaux PJ, Elbourne D, Egger M, Altman DG (March 2010). "CONSORT 2010 explanation and elaboration: updated guidelines for reporting parallel group randomised trials". BMJ. 340: c869. doi:10.1136/bmj.c869. PMC 2844943. PMID 20332511.
- ^ "Standard errors, confidence intervals, and significance tests". StataCorp LLC.
- ^ a b Szklo, Moyses; Nieto, F. Javier (2019). Epidemiology : beyond the basics (4th. ed.). Burlington, Massachusetts: Jones & Bartlett Learning. p. 488. ISBN 9781284116595. OCLC 1019839414.
- ^ Katz, D.; Baptista, J.; Azen, S. P.; Pike, M. C. (1978). "Obtaining Confidence Intervals for the relative risk in Cohort Studies". Biometrics. 34 (3): 469–474. doi:10.2307/2530610. JSTOR 2530610.
- ^ Armitage P, Berry G, Matthews JN (2002). Armitage P, Berry G, Matthews J (eds.). Statistical Methods in Medical Research. Vol. 64 (Fourth ed.). Blackwell Science Ltd. p. 1168. doi:10.1002/9780470773666. ISBN 978-0-470-77366-6. PMC 1812060.
{{cite book}}
:|journal=
ignored (help)